Radicle Science (San Diego, CA) is using an AI-powered, direct-to-consumer model that not only makes clinical trials more affordable, but also increases their speed and scale. As a result, Radicle Science is making clinical substantiation accessible to more dietary supplement manufacturers who can leverage high quality data for verifiable claims, research and development, and targeted marketing.
The Problem
Radicle Science was co-founded by Pelin Thorogood and Jeff Chen, MDas a solution to the limitations of traditional clinical trials, which are high cost and have limited data sets that do not accurately represent consumer populations. Thorogood and Chen come from significantly different professional backgrounds. Chen graduated from UCLA’s David Geffen School of Medicine and Anderson School of Management with an MD and Masters in Business Administration (MBA), respectively, with the goal of running healthcare systems.
“At the conclusion of that program, what I realized was the current ways that we’re delivering health — the way you bring the treatment to market, the way that once it’s in the market all these gatekeepers are involved, the cost that can be involved — wasn’t really going to dramatically change health in any short amount of time,” explains Chen.
Jeff Chen, MD, co-founder and CEO of Radicle Science. Image courtesy of Radicle Science
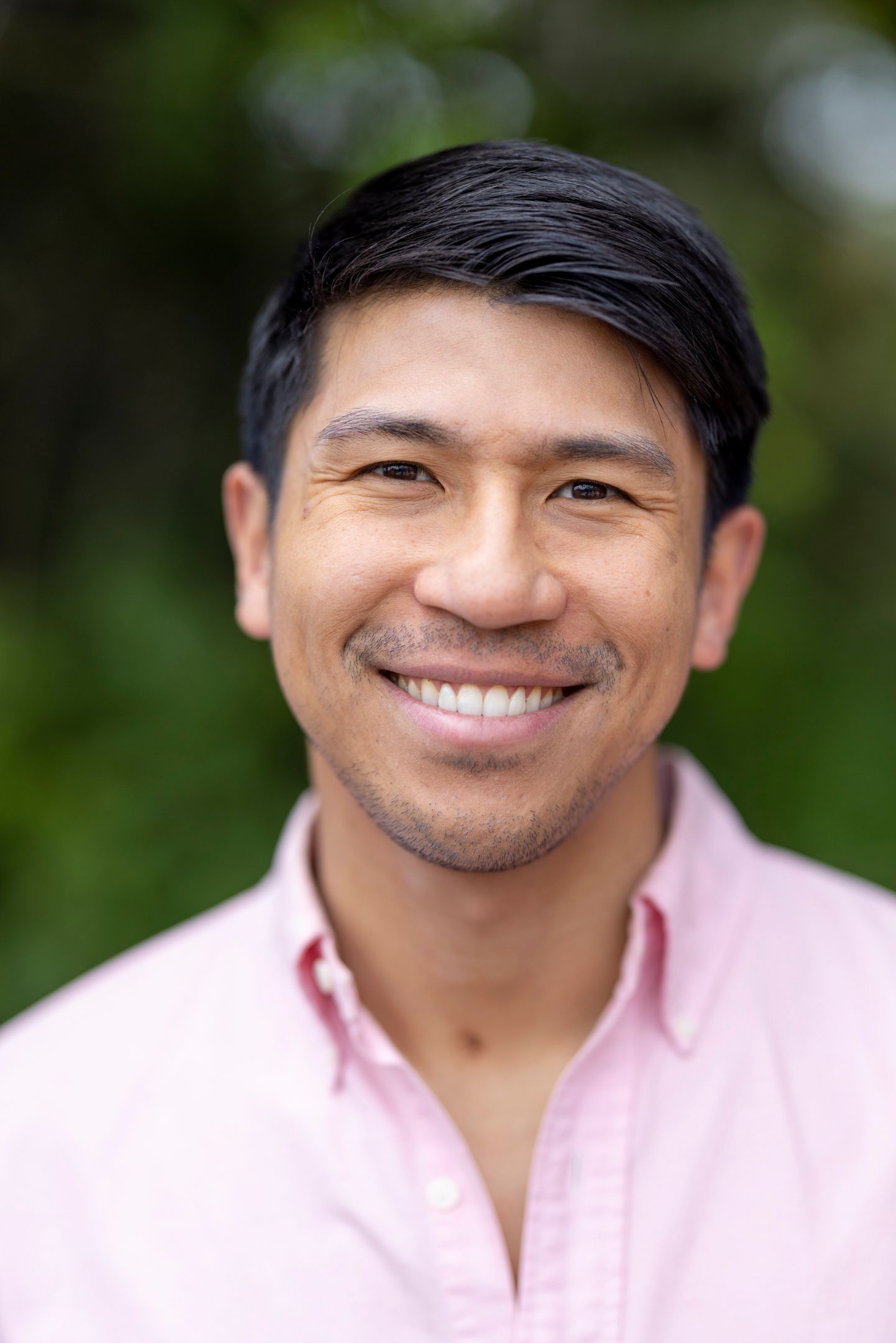
In his time living in Los Angeles and attending UCLA, Chen witnessed many people turning to non-traditional interventions such as supplements, specialized diets, and mind-body practices that challenged what he was taught in medical school, namely that these interventions had no evidence and should be ignored in favor of FDA-approved interventions. So, he began to think that if more data can be generated on these non-pharmaceutical interventions, perhaps these forms of alternative medicine can be validated and more affordable treatments can be brought to market faster.
“I started a research center at UCLA after med school focused on running clinical trials on botanical products in an effort to raise the credibility of them, but came face to face with how slow and expensive the traditional process was, as well as many things that still were lacking in the data sets that we were creating,” says Chen. Along the way he met Thorogood.
Thorogood’s background is in data and analytics. She studied operations research information engineering at Cornell University where she received her undergraduate and graduate degrees, as well as an MBA. Since then, Thorogood has achieved a great deal of success in the world of tech. She was previously chief marketing officer of WebSideStory, one of the pioneers in marketing technology that was acquired by Adobe. She went on to become CEO of Anametrix, one of the earliest predictive analytics companies. That company was later acquired by Ensighten. It was a family health crisis that spurred her interest in healthcare and clinical trials. Thorogood became a philanthropist, co-founding the Wholistic Research and Education Foundation, which was dedicated to the research of CBD and cannabis-based therapeutic solutions.
Pelin Thorogood, co-founder and executive chairwoman of Radicle Science. Image courtesy of Radicle Science.
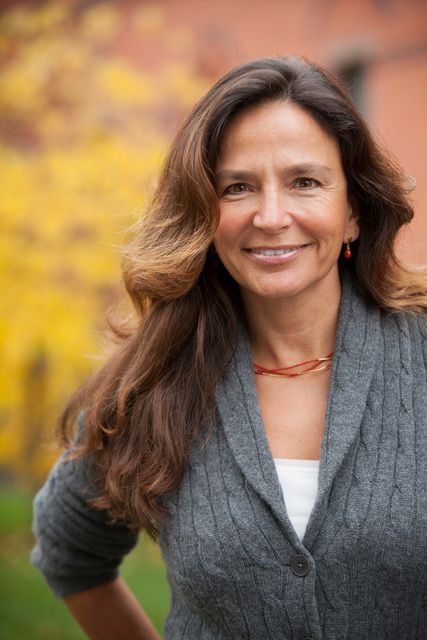
“What I realized during that time were a few things: A) how expensive and how slow these clinical trials are. We were funding them in some of the most amazing research universities in the country and they’re doing an incredible job, but that’s just a traditional system, the system that was designed for pharma. The other part that was really obvious to me was how small these data sets were. A lot of these massive multi-million dollar clinical trials were conducted on dozens of people, not hundreds or thousands of people, and they were homogeneous. Because of the small data sets, to actually have predictive power we’re looking at similar populations…and really not providing diversity,” says Thorogood. “So, I was realizing we were not going to get the generalizability that you could get from a large data set to have that predictive power that could enable the data to be useful for the population at large.”
With similar frustrations about the existing clinical trial models and complementary backgrounds, Thorogood and Chen teamed up on Radicle Science in the wake of the COVID-19 pandemic, during which clinical trials came to a complete halt. This created an opportunity for novel protocols that accommodated the limitations of lockdowns and social distancing.
The Solution
One of the major advantages of the Radicle Science model, say Thorogood and Chen, is the ability to study large (minimum of 500 participants) and diverse populations. This is made possible by Radicle Science’s Proof-as-a-Service technological platform thatprovides end-to-end automation of its clinical trial process. Remote and direct-to-consumer, Radicle Science trials don’t require in-person data collection that limits populations to specific geographical regions. A more diverse population translates to a more representative population. The convenience of the remote trials also makes compliance easier, reducing their dropout rates.
“As a result, we have gender parity in our study populations, we have well over 20% ethnic populations participation. We have rural populations over 20% participating who may have different exercise, have different dietary habits, so we’re bringing not just DEI into it,” says Thorogood. “With different types of health demographics as well as behavioral elements, our data are more representative of the population.”
Chen also emphasizes that beyond being more representative of the population, the data collected by Radicle Science is more representative of the real-world impacts of the products, compared to the more controlled laboratory environment. “For us to collect data from people in the naturalistic setting, in their own home, in their day-to-day [life], whether we’re collecting digital information, whether we’re sending kits for biomarkers, those outcomes are much more predictive of how these products will actually be delivering effects when they’re purchased off a shelf and used in the real world,” explains Chen.
Through its use of AI, Radicle Science is also able to take these huge datasets and extract multidimensional data. “Looking at multiple dimensions in terms of demographics, lifestyles, behavior, and leveraging AI on these massive data sets gives us not just a statistical average as to whether this [product] works on average for the non-existent statistical person, but how it may actually work for different consumer segments, subpopulations,” says Thorogood. “So, AI becomes highly predictive and then you leverage statistics to validate and make sure that there’s clinical significance behind those predictions as well. So, it’s a beautiful dance of using AI, having the hypotheses and then using statistics on those new hypotheses to be able to validate and then use in a clinical setting.”
Chen adds that on top of the scale of the studies, and the application of machine learning, their studies also have various levels of control implemented such as blinding, randomization, and placebo-control that add to the quality of the data.
Implementation
Radicle Science has standardized master protocols for substantiating popular health benefits such as pain, sleep, stress, gut health, and menopause, for example. However, the company also offers configurability into these standardized offerings that allow manufacturers to explore specific age groups or genders, and specify different outcomes that may better meet their needs. Chen points out that because of the large population sizes used by Radicle Science, the studies are also powered to look at multiple health outcomes. That means that a manufacturer may do a trial focused on sleep, but may come away from the study with multiple distinct claims that relate to stress, mood, and quality of life, for example.
Harkening back to the idea of multidimensional data, the size and diversity of the study population also gives manufacturers an opportunity to learn how their products impact distinct subpopulations.
“Because we have run so many trials and have included so many participants with such diversity, we see that clearly there are distinct subpopulation effects of these products,” explains Chen. While many brands may think their products are beneficial across the board, that may not always be the case. There may be distinct gender and age-group differences, for example, or even different impacts depending on the severity of a condition. “We can allow the client to hone their eligibility criteria to just focus on that subpopulation for the whole trial, which would theoretically increase their chances of statistical significance,” says Chen.
The studies may also aid in research and development through the use of multi-arm parallel group trials. “They could try five different formulas or it could be a couple of distinct formulations and then a double dose of those formulations and those will all be parallel arms that would just be studied simultaneously in the trial,” explains Chen. “What you find that comes out of it is maybe you have multiple formulations that had statistical significance, but one only had a statistical significance in one health area, while one had multiple health areas. Now you can compare the cost structure to determine which one is the most efficient product to launch.”
“We all know that there’s a churn issue in many of the dietary supplements because people are trying multiple things. So, if you’re product is more likely to work, your acquisition dollars are going to go towards people that are likely to benefit, who are likely to repeat purchase, so it actually is going to provide long term benefits from a growth and revenue and profits perspective,” added Thorogood. “This is the kind of data that can inform innovation. It can inform claims that are very rigorous and definitely keep you on the safe side as well as informed marketing so that you’re marketing to the right populations as well as providing this kind of data to your retail partners.”
This post was originally published on here